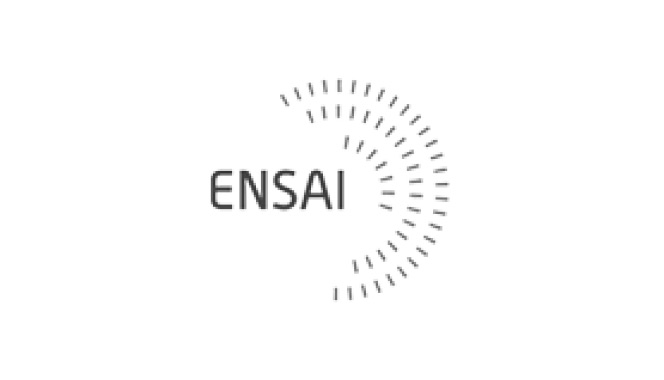
Modelling of the impact of physical and transition risk on banks’ solvency
Climate risk has now been clearly identified as a risk to be taken into account in banks’ capital requirements.
22 September 2023 | Adway, Risque & Finance, Square Research Center
Climate risk has now been clearly identified as a risk to be taken into account in banks’ capital requirements.